As the healthcare industry continues to evolve, the integration of artificial intelligence (AI) has become increasingly prevalent. In this article, we delve into the top AI in healthcare books that offer insights into the future of medicine, exploring various applications, ethical considerations, challenges, opportunities, and the impact on patient care and outcomes.
Key Takeaways
- AI in healthcare presents a wide range of applications from diagnostics to treatment planning and drug discovery.
- Ethical considerations such as privacy, bias, and transparency are crucial in AI-driven healthcare.
- Challenges in implementing AI include regulatory compliance, system integration, and workforce training.
- AI in healthcare enables personalized medicine, remote monitoring, and predictive analytics for improved patient care and outcomes.
- Understanding the implications of AI in healthcare is essential for navigating the future of medicine and ensuring positive impacts on patient well-being.
Exploring AI Applications in Healthcare
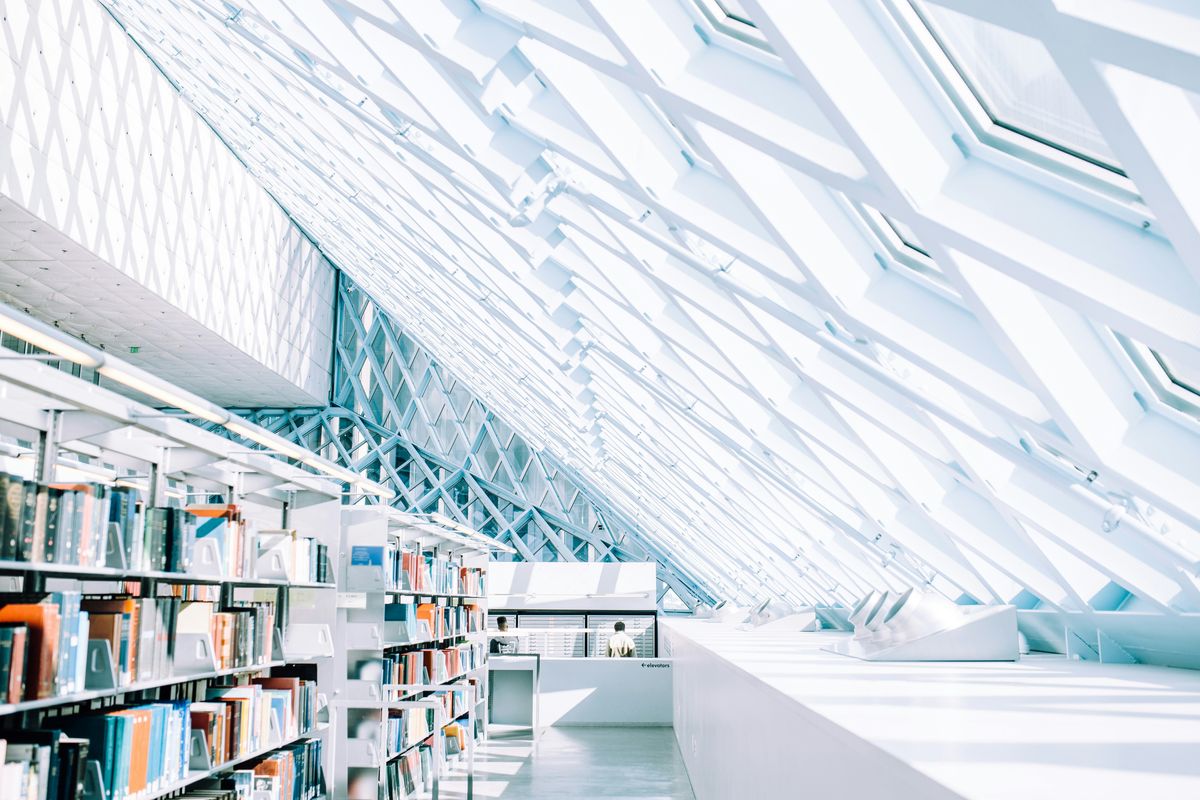
AI in Diagnostics
The integration of Artificial Intelligence (AI) in diagnostics is revolutionizing the way medical professionals identify and understand diseases. AI algorithms are now capable of analyzing complex medical data at unprecedented speeds, often with greater accuracy than traditional methods. This capability is particularly transformative in the field of medical imaging, where AI can detect patterns invisible to the human eye.
Early detection is crucial in improving patient outcomes, and AI is instrumental in achieving this by enabling the analysis of vast datasets to identify potential health issues before they become critical. For instance, AI systems can sift through millions of radiology images to spot minute signs of cancerous growths, often at an early stage when treatment is more likely to succeed.
- Enhanced precision in diagnosis
- Reduction in diagnostic errors
- Accelerated diagnostic processes
The potential of AI to support diagnostic professionals and reduce the burden of manual analysis is immense, paving the way for more efficient healthcare delivery.
However, the adoption of AI in diagnostics also necessitates rigorous validation to ensure the reliability of these systems. It is essential that healthcare providers and patients alike can trust the accuracy and consistency of AI-driven diagnoses.
AI in Treatment Planning
The integration of Artificial Intelligence (AI) into treatment planning is revolutionizing the healthcare industry. AI algorithms are now capable of analyzing complex medical data to assist in creating personalized treatment plans for patients. This not only enhances the precision of treatments but also significantly reduces the time required for healthcare professionals to devise effective strategies.
Predictive modeling, a key aspect of AI, enables the anticipation of treatment outcomes, allowing for adjustments before implementation. This proactive approach can lead to improved patient care and more efficient use of resources.
The potential of AI to transform treatment planning is immense, offering a future where medical interventions are tailored to the individual needs of each patient.
Challenges such as data quality and algorithmic reliability must be addressed to fully harness AI's capabilities in treatment planning. However, the opportunities for improved patient outcomes and streamlined healthcare processes are substantial.
- Integration of AI with patient data
- Development of personalized treatment plans
- Continuous learning and adaptation of AI systems
- Collaboration between AI experts and healthcare professionals
AI in Drug Discovery
The integration of Artificial Intelligence (AI) in drug discovery is revolutionizing the pharmaceutical industry. AI algorithms can analyze vast datasets to identify potential drug candidates much faster than traditional methods. This acceleration in the early stages of drug development is crucial for responding to emerging health threats and chronic diseases.
Machine learning models are particularly adept at predicting molecular behavior, which can lead to the discovery of new drugs with fewer side effects. These models can also simulate clinical trials, reducing the need for costly and time-consuming physical trials.
- Identification of novel drug candidates
- Acceleration of drug-to-market timelines
- Reduction of development costs
- Enhancement of drug efficacy and safety
The potential of AI to transform drug discovery extends beyond mere efficiency. It promises to unlock new therapeutic possibilities and tailor treatments to individual genetic profiles, heralding a new era of personalized medicine.
Ethical Considerations in AI-Driven Healthcare
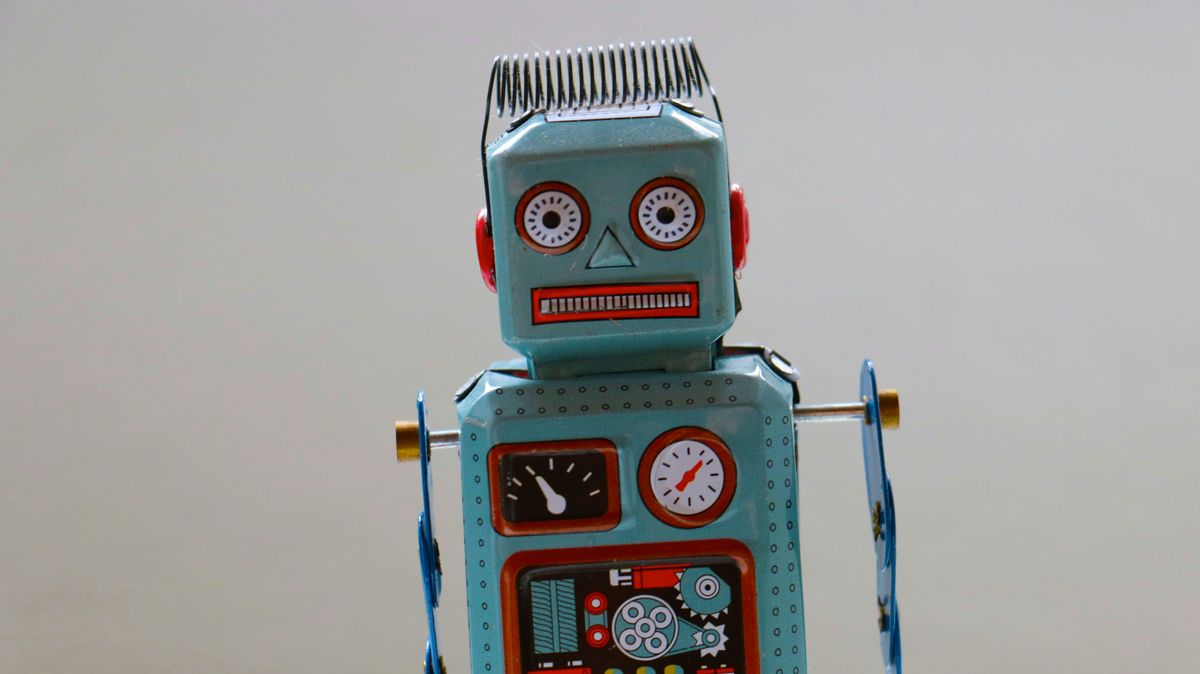
Privacy and Data Security
In the realm of AI-driven healthcare, privacy and data security are paramount. The vast amounts of sensitive patient data necessitate robust protection mechanisms to prevent breaches that could have severe consequences. Healthcare providers must ensure compliance with regulations such as HIPAA and GDPR, which are designed to safeguard patient information.
- Development of secure data storage solutions
- Implementation of end-to-end encryption
- Regular security audits and updates
- Training staff on data privacy best practices
The integration of AI in healthcare brings the challenge of maintaining patient confidentiality while leveraging data for improved care. Balancing these priorities requires a thoughtful approach to data governance and the use of advanced security technologies.
Bias and Fairness
The integration of AI in healthcare brings to the forefront critical issues of bias and fairness. Ensuring equitable AI systems is paramount to maintain trust and effectiveness in medical applications. Bias in AI can arise from various sources, often stemming from the data used to train algorithms. If the data is not representative of the diverse patient populations, the AI system may perform ineffectively for underrepresented groups, leading to disparities in healthcare outcomes.
- Historical biases in healthcare data
- Lack of diversity in clinical trials
- Algorithmic biases due to training data
It is essential to implement rigorous testing and validation processes for AI systems to identify and mitigate biases. This includes continuous monitoring and updating of AI models to reflect the evolving demographics and needs of the patient population.
Healthcare organizations must collaborate with diverse stakeholders, including ethicists and patient advocacy groups, to develop guidelines that ensure AI systems are fair and just. This collaborative approach can help in establishing standards for transparency and accountability in AI-driven healthcare solutions.
Transparency and Accountability
In the realm of AI-driven healthcare, transparency is crucial for building trust between patients and healthcare providers. It involves clear communication about how AI systems make decisions and the factors that influence these decisions. Similarly, accountability ensures that there is a clear assignment of responsibility for the outcomes of AI interventions.
- Clear guidelines for AI system transparency
- Defined protocols for accountability in AI outcomes
- Regular audits of AI systems to ensure compliance
Ensuring transparency and accountability in AI-driven healthcare not only fosters trust but also promotes a culture of continuous improvement and ethical responsibility.
While transparency allows patients to understand the AI-driven processes affecting their care, accountability is essential for maintaining the integrity of healthcare services. Together, they form the bedrock of ethical AI use in medicine.
Challenges and Opportunities in Implementing AI in Healthcare
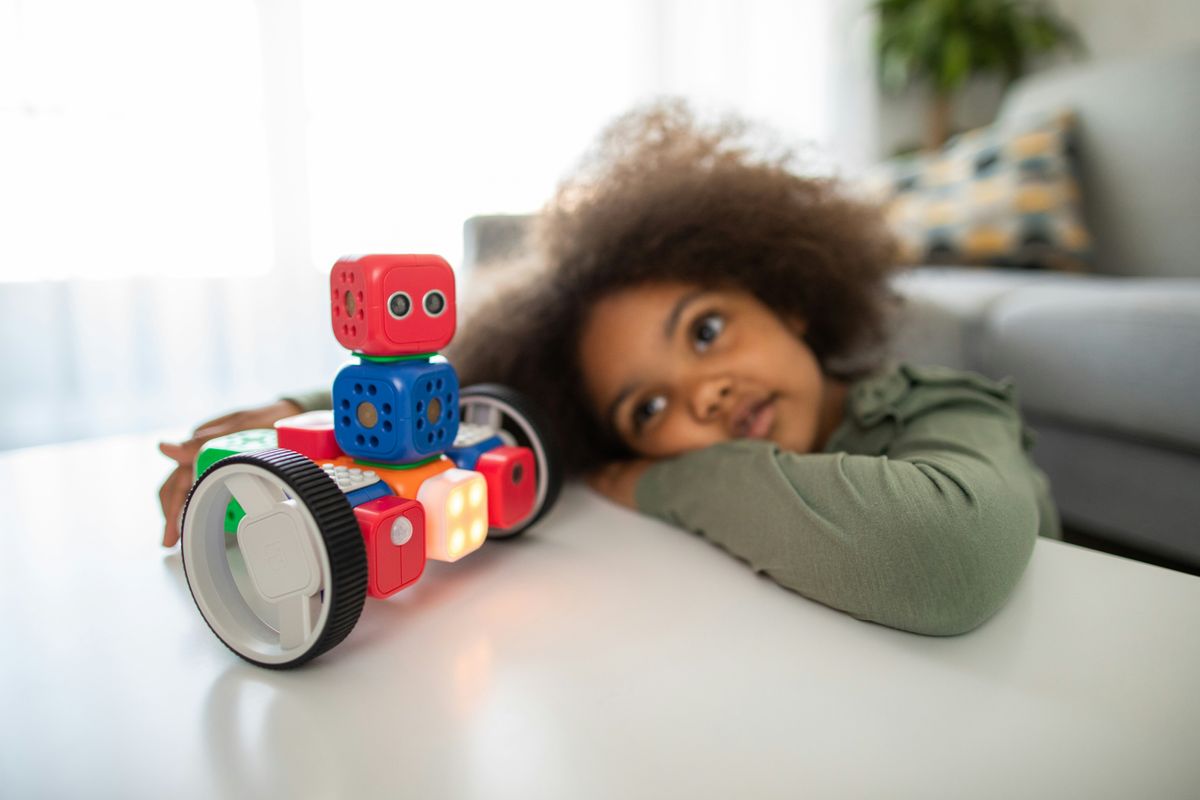
Regulatory Compliance
The integration of Artificial Intelligence (AI) in healthcare is subject to stringent regulatory compliance to ensure safety, efficacy, and ethical standards. Navigating the complex landscape of regulations is a critical step for healthcare providers and AI developers alike. The Food and Drug Administration (FDA) in the United States, for example, has established guidelines for AI-based medical devices, which must undergo rigorous review processes before they can be deployed in clinical settings.
- Understanding the regulatory framework
- Adhering to international standards like HIPAA and GDPR
- Ensuring continuous compliance through monitoring and reporting
The dynamic nature of AI technology poses unique challenges for regulators, who must balance the need for innovation with public health and safety concerns. As AI systems evolve, so too must the regulations that govern them, requiring ongoing dialogue between technologists, healthcare professionals, and policymakers.
Integration with Existing Systems
The successful integration of AI technologies into existing healthcare systems is a critical step towards a more efficient and effective medical infrastructure. Seamless integration ensures that AI tools enhance, rather than disrupt, the workflows of healthcare professionals. One of the main challenges is the compatibility of AI solutions with legacy systems, which often requires significant technical adjustments.
Interoperability is a key factor in the integration process, as it allows for the secure and efficient exchange of data between different software applications and hardware systems. To achieve this, healthcare organizations often need to follow a series of steps:
- Assessment of current IT infrastructure
- Identification of potential integration points
- Development of a strategic plan
- Execution of integration with continuous testing and feedback
The goal is to create a cohesive ecosystem where AI tools not only coexist with traditional systems but also complement and enhance the capabilities of healthcare providers. This requires a thoughtful approach that balances innovation with practicality, ensuring that patient care remains the top priority.
Healthcare Workforce Training
The integration of AI into healthcare necessitates a paradigm shift in workforce training. Healthcare professionals must be equipped with the necessary knowledge and skills to effectively collaborate with AI systems. This includes understanding the capabilities and limitations of AI, as well as the ethical implications of its use in clinical settings.
Education and training programs need to be updated to include AI-related competencies. These programs should be designed to cater to a variety of roles within the healthcare sector, from physicians and nurses to administrative staff and IT professionals. A multi-disciplinary approach is essential for fostering an environment where AI can be used safely and effectively.
- Identify core AI competencies for healthcare roles
- Develop tailored training modules for different specialties
- Establish continuous learning and development opportunities
The successful adoption of AI in healthcare hinges on the readiness of its workforce to adapt to new technologies. Ensuring that healthcare professionals are well-prepared will not only enhance the integration of AI but also improve patient care and outcomes.
Impact of AI on Patient Care and Outcomes
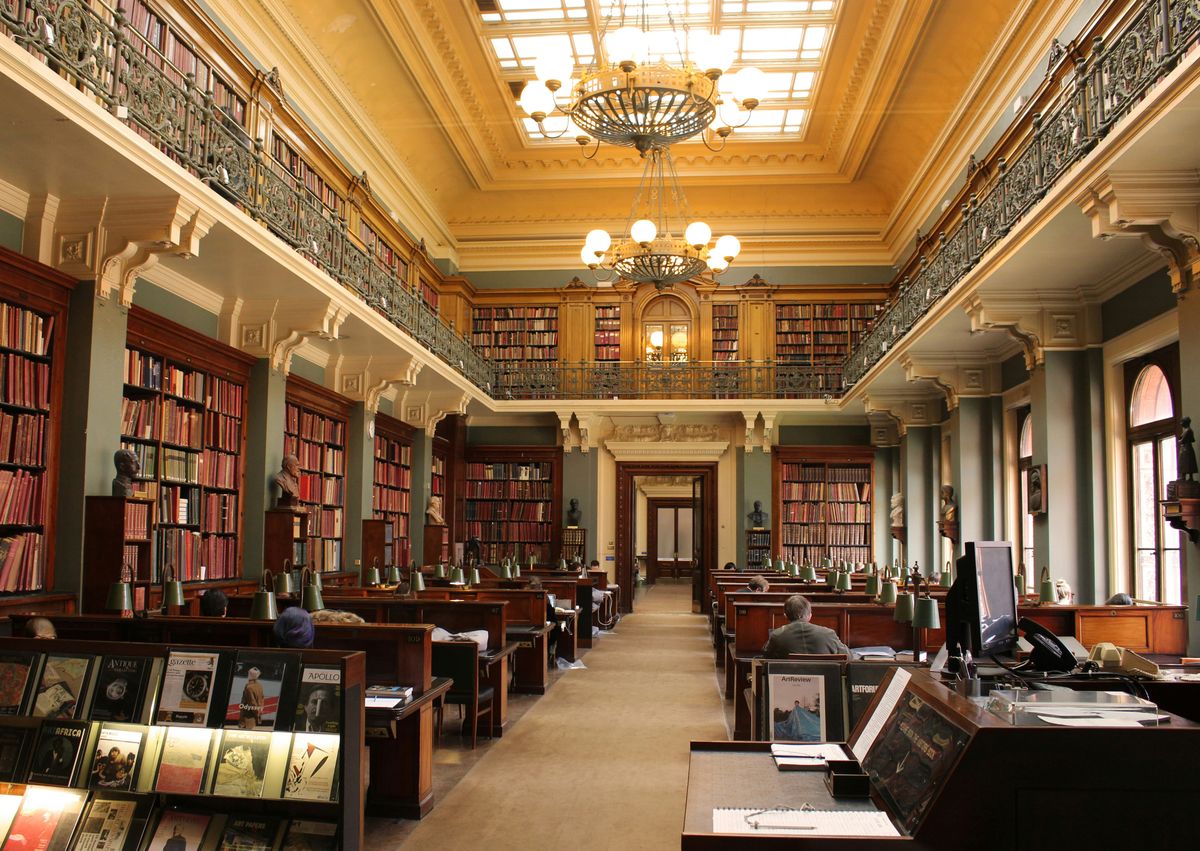
Personalized Medicine
The advent of AI in healthcare has paved the way for personalized medicine, tailoring treatment to the individual characteristics of each patient. AI algorithms analyze vast datasets, including genetic information, to identify the most effective treatments for specific conditions.
- Identification of genetic markers linked to diseases
- Analysis of patient's unique genetic profile
- Customization of treatment plans
The precision of personalized medicine offers the potential to significantly improve patient outcomes by targeting therapies to individuals' unique genetic makeup. This approach minimizes the trial-and-error process often associated with traditional treatments, leading to more efficient and effective care.
However, the implementation of personalized medicine also requires careful consideration of ethical and privacy concerns, as sensitive genetic data is integral to the process.
Remote Monitoring
The advent of AI in healthcare has revolutionized the concept of remote monitoring, enabling continuous patient care beyond the confines of medical facilities. Remote monitoring systems, powered by AI, can now predict acute medical events before they occur, allowing for timely interventions. These systems collect a myriad of data points, from heart rate to activity levels, providing a comprehensive view of a patient's health status.
Telehealth has become a critical component in managing chronic diseases, where regular monitoring is essential. AI-driven remote monitoring tools have shown to improve patient engagement and adherence to treatment plans, as they feel more connected to their healthcare providers.
- Enhanced patient convenience
- Reduced hospital readmissions
- Increased healthcare accessibility
The integration of AI into remote monitoring represents a significant stride towards a more proactive and preventive healthcare approach. It underscores the potential of AI to not only support healthcare professionals but also empower patients in managing their health.
Predictive Analytics
The advent of predictive analytics in healthcare is transforming the way clinicians anticipate and address patient needs. By analyzing vast datasets, AI algorithms can identify patterns that may indicate the likelihood of future medical events, such as hospital readmissions or the onset of chronic diseases. This predictive capability enables proactive interventions, potentially improving patient outcomes and reducing healthcare costs.
Predictive analytics also plays a crucial role in resource allocation within healthcare facilities. By forecasting patient admissions, hospitals can optimize staffing levels and equipment availability, ensuring that resources are appropriately allocated to meet anticipated demand. The following table illustrates the impact of predictive analytics on hospital readmission rates:
Year | Readmission Rate (Before) | Readmission Rate (After) |
---|---|---|
2019 | 15.2% | 14.1% |
2020 | 14.8% | 13.5% |
2021 | 14.3% | 12.9% |
The integration of predictive analytics into clinical practice is not without challenges. It requires a robust digital infrastructure, skilled personnel to interpret the data, and continuous algorithm refinement to maintain accuracy over time.
As healthcare systems continue to evolve, the role of predictive analytics will likely expand, offering new ways to enhance patient care and streamline operations.
Conclusion
In conclusion, the field of AI in healthcare is rapidly evolving, offering immense potential to revolutionize the way medicine is practiced. The books highlighted in this article provide valuable insights into the current landscape and future possibilities of AI in healthcare. As technology continues to advance, it is crucial for healthcare professionals to stay informed and adapt to these changes. By embracing AI technologies responsibly and ethically, we can navigate the future of medicine with innovation and compassion.
Frequently Asked Questions
What are the key AI applications in healthcare?
AI applications in healthcare include diagnostics, treatment planning, and drug discovery.
How can AI-driven healthcare ensure privacy and data security?
AI-driven healthcare can ensure privacy and data security through encryption, access controls, and compliance with regulations like HIPAA.
What are the ethical considerations in AI-driven healthcare?
Ethical considerations in AI-driven healthcare include issues related to privacy, bias, transparency, and accountability.
What challenges are faced in implementing AI in healthcare?
Challenges in implementing AI in healthcare include regulatory compliance, integration with existing systems, and healthcare workforce training.
How does AI impact patient care and outcomes?
AI impacts patient care and outcomes through personalized medicine, remote monitoring, and predictive analytics.
What opportunities does AI present in healthcare?
AI presents opportunities in healthcare such as improved diagnostics, personalized treatment plans, and enhanced patient outcomes.